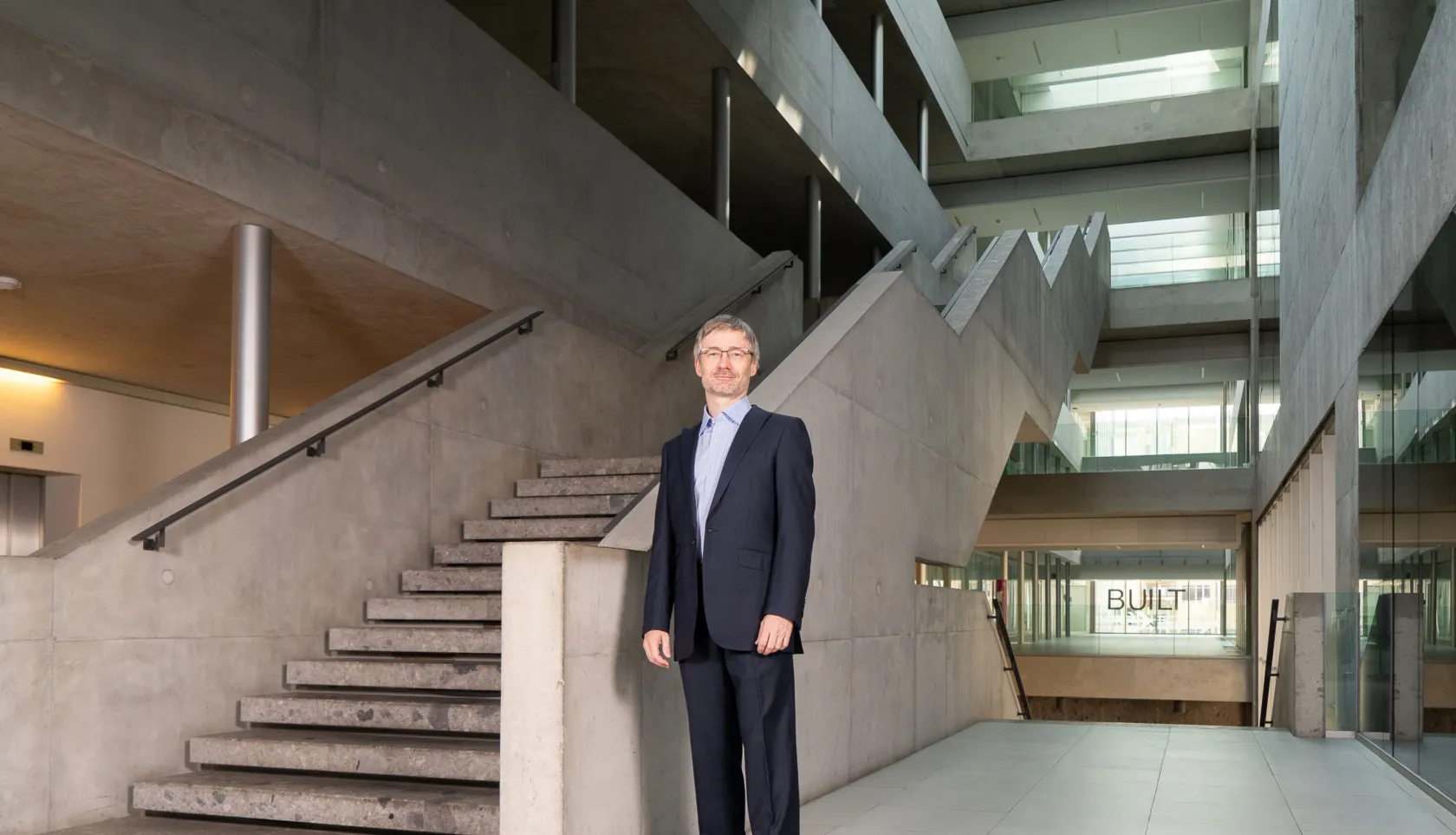
When the Future Feels Fuzzy
Imagine you’re packing for a weekend trip, but the weather forecast is unclear. You make a plan—but halfway through the drive, the outlook changes. Do you stick to your plan or change course?
This simple dilemma captures a deep question in economics: how should we make decisions under uncertainty, and how should those decisions adapt as new information arrives?
Economists want decision models that are smart, consistent over time, and able to handle ambiguity—not just risks whose probability distributions are known. A new paper published in the Journal of Economic Theory by Andrei Savochkin (Bocconi Department of Decision Sciences), Alexander Shklyaev, and Alexey Galatenko (both of Moscow State University) tackles this challenge, focusing on one of the most sophisticated tools in the field: the Smooth Ambiguity model.
From risk to ambiguity
In classic decision theory, uncertainty is treated as risk—you know the odds. But real life often deals with ambiguity: even the probabilities associated to possible scenarios are unknown. Think of trying to predict future AI impacts or election outcomes: the problem isn’t just what might happen, but how unsure we are about how unsure we are.
The Smooth Ambiguity model captures this two-layered doubt. It is a way of describing how people make decisions when they face not just uncertainty, but ambiguity. Instead of assuming one fixed view of how likely different outcomes are, the model allows for multiple possible scenarios. People first imagine how good or bad each outcome would be under each scenario, then weigh those impressions using a second layer of judgment that reflects their attitude toward ambiguity. If they dislike ambiguity, they'll associate higher weight to scenarios that feel more reassuring.
The model captures both how people evaluate risks and how they feel about not knowing which risks they’re really facing, and works reasonably well in static situations—but what happens when information unfolds over time?
The dynamic consistency puzzle
In a dynamic world, good decisions should follow two rules:
- Dynamic consistency: if a plan was expected to be the best given the possibility of certain news, it should still be chosen when that news actually arrives.
- Consequentialism: decisions should depend only on outcomes that still matter, not on those that have been ruled out by the newly received information.
These rules hold in standard models of risk. But ambiguity makes things trickier.
For a simpler model (called “maxmin”), earlier research found that you can preserve both rules by organizing beliefs in a very precise way—something called rectangularity. But whether Smooth Ambiguity could do the same was an open question.
New insight
So, can the Smooth Ambiguity model—this flexible, realistic way of representing uncertainty—actually work in a dynamic setting, where choices unfold over time?
The short answer is: yes, but only under fairly strict conditions. The authors’ contribution is to show exactly what those conditions are. First, they prove that if there is no ambiguity at one point in the decision process—say, you’re sure about what happens in the first or second stage—then things are fine. But that’s not very satisfactory, because ambiguity is the whole point of the model.
The more interesting case is when ambiguity is present throughout. In that case, they show that only a special kind of ambiguity attitude—called constant absolute ambiguity aversion—will do the trick. In plain terms, this means the decision-maker dislikes ambiguity in a consistent, mathematically smooth way across all scenarios.
What’s more, even under this condition, the person’s beliefs about possible outcomes have to follow a specific pattern—one that allows you to break down decisions recursively, as if you were folding the problem backwards step by step. This structure, though technically more complex than the “rectangularity” condition from earlier work, plays a similar role: it ensures that future decisions can be built from present ones without contradictions.
In other words, Smooth Ambiguity preferences can behave well over time, but only if the model is carefully structured. You can’t just drop ambiguity into a dynamic setting and expect it to work. You need to shape the model in the right way—mathematically and conceptually.
The paper thus defines the narrow but usable pathway for applying Smooth Ambiguity to dynamic problems. And for economists or policymakers who want to use this model in real-life forecasting, it’s an essential bit of guidance.