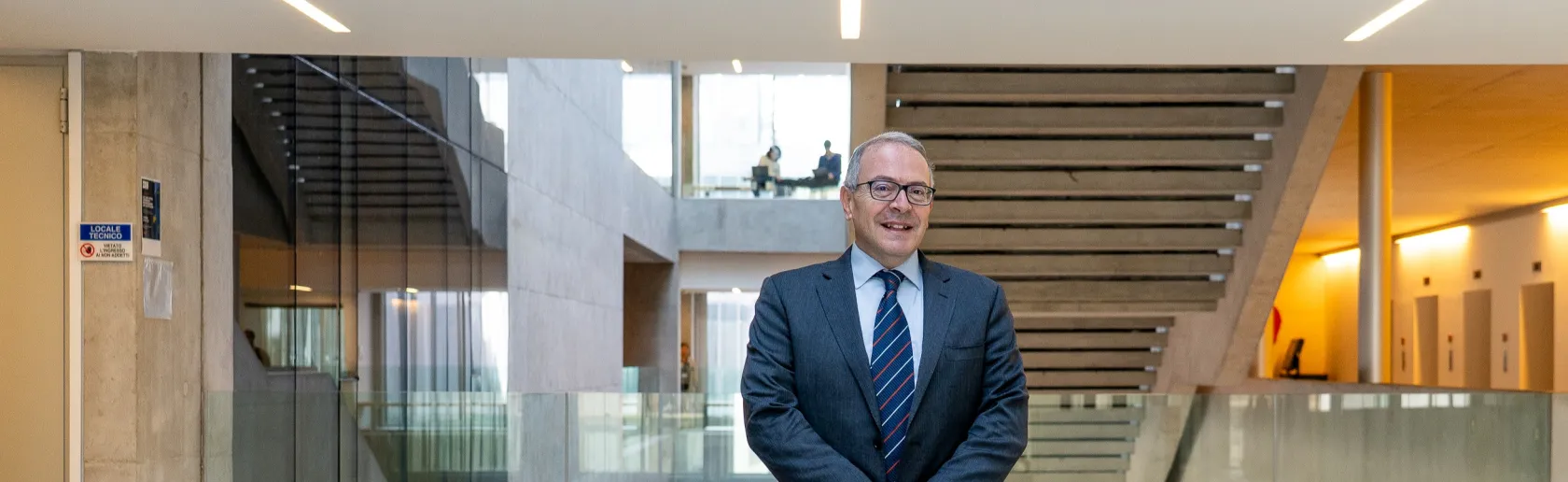
When Finance Strikes, the Economy Does Not Always React the Same Way
What happens to the U.S. economy when the financial system is rocked by a shock? The answer today is neither linear nor symmetric. Negative shocks — tightening credit or increasing perceived risk — induce much stronger macroeconomic reactions than positive ones. But there is more: what changes is not so much the intensity of the shock as its sign. Whether the shock is small or large, if it is adverse it still triggers strong and persistent responses on inflation, employment and industrial production.
These findings emerge from a study published in Economics Letters (vol. 250, April 2025), entitled Machine learning the macroeconomic effects of financial shocks. The authors, Niko Hauzenberger (University of Strathclyde), Florian Huber (University of Salzburg), Karin Klieber (Österreichische Nationalbank) and Massimiliano Marcellino (Bocconi)-propose an innovative approach that combines structural econometrics with Bayesian Neural Networks (BNN), an artificial intelligence tool particularly well suited to capture nonlinearities in economic data.
“The real methodological innovation,” explains Marcellino, director of Bocconi's Baffi Research Center, “is that we can use machine learning techniques to study impulse responses without having to specify a functional form in advance. This approach allows us to detect hidden dynamics, such as asymmetries in the signs of responses, that often escape traditional linear models.”
In detail, the method uses BNNs to estimate local nonlinear projections, calculating the expected effect of a financial shock-as measured by the excess bond premium on three key variables: inflation (CPI), industrial production (INDPRO) and employment (CE16OV). The results speak for themselves: in the event of a negative shock, inflation falls sharply (by up to one percentage point), industrial production falls by almost two points, and employment contracts for two years. The same effects are not observed in the presence of positive shocks: there is little, sometimes no reaction.
“Negative shocks have a much more pronounced macroeconomic impact than positive shocks of similar magnitude,” Marcellino points out. “And this is true for inflation as well as industrial production and employment.”
The study is also notable for a robust simulation exercise on artificially generated data from highly nonlinear processes. Bayesian neural networks, in this context, manage not only to faithfully recover expected responses, but also to distinguish between sign and size asymmetries. A result that confirms the model's ability to accurately capture the complexity of macroeconomic dynamics.
“This kind of evidence has crucial implications for monetary policy and financial stability,” Marcellino concludes. “If we know that the economic system is much more sensitive to negative shocks, then it is critical to act early with preventive tools, rather than waiting for the crisis to erupt.”
In an increasingly uncertain world, in which predictive models must contend with complex, nonlinear dynamics, tools such as Bayesian Neural Networks represent a promising frontier: capable not only of predicting, but also of explaining.
How the model works: inside Bayesian neural networks
The approach is based on a Bayesian Neural Network (BNN) with a hidden layer composed of a number of neurons equal to the number of macroeconomic covariates. Each neuron can use a different activator function: leaky ReLU, sigmoid, ReLU, and tanh. BNNs combine these activators with specific weights to generate flexible but interpretable responses.
To avoid overfitting due to the large number of parameters, the authors use a regularization system called horseshoe prior, which “squashes” toward zero the irrelevant coefficients, keeping only those that are essential for explaining the phenomenon.
Another distinguishing feature is the management of uncertainty about latent shocks. Instead of treating errors as fixed residuals, the model adopts a sequential Bayesian approach, estimating step by step the distribution of shocks at different time horizons.
Finally, nonlinear local projections (NLP) are computed not on a single scenario, but as an average over 400 simulated economic history trajectories (for each Markov Chain Monte Carlo draw), resulting in an estimate that is not conditional on past shocks but is robust and generalizable.