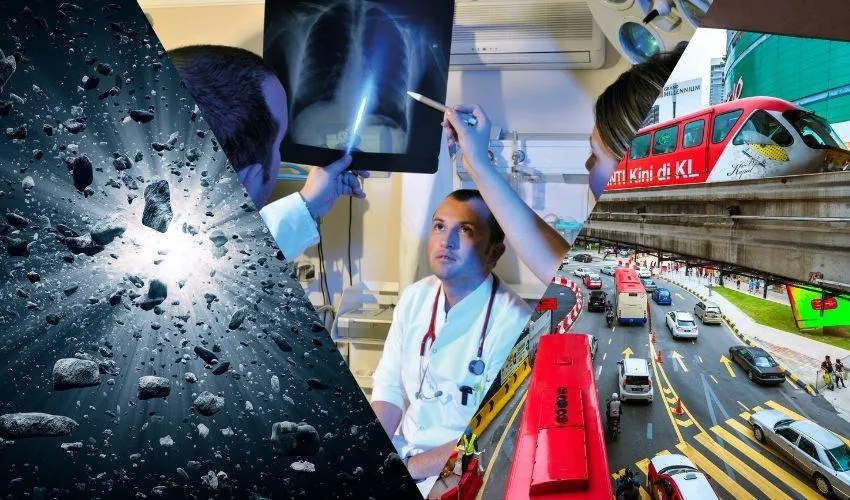
Understanding Uncertainty in Machine Learning Algorithms
If we want to understand when the universe was born and when it will end, we make use of the Hubble Constant, a measure of the current expansion rate of the universe. The Hubble Constant is calculated with statistical methods that produce both an estimate and an error range (a measure of uncertainty). The only problem: the statistical methods currently used to estimate the Constant, provide contradictory results and error ranges that don't even overlap.
"Either the underlying physics is wrong, or the statistical methods could be improved," says Botond Szabo. An Associate Professor at Bocconi Department of Decision Sciences, Szabo obtained a €1.5mln ERC Starting Grant for a project (BigBayesUQ - The missing mathematical story of Bayesian uncertainty quantification for big data) aimed at developing mathematical techniques capable of assessing the uncertainty inherent in the estimates derived from machine learning algorithms and, consequently, their reliability in context of statistical models. Such algorithms are conceived to be used when a large quantity of data is involved (as in the example of the Hubble Constant and in many other cases and disciplines).
In the world of big data, when there's the need to estimate many parameters in very complex models making use of the large amount of available information, computation time becomes unsustainable. Several shortcuts have thus been experimented with in recent years, in the form of machine learning algorithms capable of speeding up the process. The evidence seems, however, to suggest that the results of these algorithms are not always reliable.
"The real issues arise when we underestimate the uncertainty of the procedure," Professor Szabo says. "Overconfidence could cause big problems when you interpret medical images or engineer a self-driving car, two of the fields where such algorithms are used."
With his ERC-winning project, Prof. Szabo wants to understand when uncertainty is correctly measured and when it is not, in order to provide the machine learning methods with statistical guarantees. "I look at machine learning techniques from a statistical and mathematical perspective and investigate their fundamental properties in statistical models," he says. "Many machine learning algorithms are currently considered black boxes, capable of obtaining results in not-well-understood ways. I will try to open the black box and understand whether its workings are reliable."
In his work, Prof. Szabo will focus mainly – but not exclusively – on a class of statistical models, called Bayesian models. "The advantage of Bayesian models in the scope of this project," he says, "is that their results already come with a built-in measure of uncertainty."
"I joined Bocconi in September 2021," he concludes, "and I'm happy to be part of a scientifically strong environment such as the Department of Decision Sciences. Its internationally leading Statistics and Data Science group has expertise ranging from the mathematical foundations to algorithms and with strong links to various applications. This represents an ideal environment for my project."
Uncertainty in Machine Learning
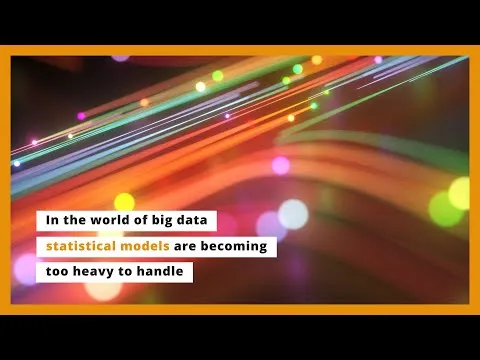