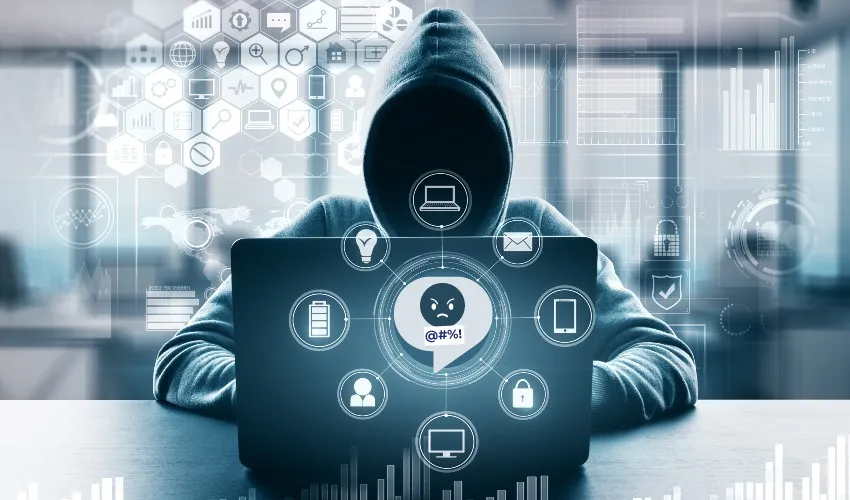
Machines Get It Wrong: How to Avoid that Woman and Gay Are Mistaken as Bad Words
In her research, Debora Nozza works to ensure that machine learning tools designed to identify and remove from social media offensive language toward minorities (the so-called "hate speech") do not end up restricting those same minorities' freedom of expression.
The LGBT+ community, like other religious, ethnic, etc. minorities, is often the victim of verbal attacks on social media. In an attempt to limit this phenomenon, given the increasing amount of posts and messages published every day, automated tools capable of detecting it have been developed. "These machine learning tools work very well in the restricted test environment," says Dr. Nozza, Postdoctoral Research Fellow at Bocconi's Department of Computing Sciences, "but face issues in the real world: in particular, the words that identify victims of attacks can end up being classified as offensive."
To train a machine learning system to detect online hate, we normally use a large set of examples containing offensive and non-offensive phrases (the so-called training set), and let the system "learn" what features make a phrase insulting. During training, however, we can expect many homophobic sentences to contain, for example, the word "gay" and many misogynistic sentences the word "woman." For this reason, it may happen that the system learns to consider the words "gay" and "woman", and all sentences containing them, as offensive. If detection were to be automatically followed by deletion, an expression such as "what a brilliant woman" would be deleted, as would the "Gay Pride" announcement posted by an LGBT+ association.
In most cases, such a bias is mitigated by feeding the system a list of words, identified by a human operator, not to be classified as offensive. "Language, however, is constantly evolving," Dr. Nozza explains, "with the creation, or increasing prevalence of terms related, for example, to gender identity. In these cases, a system that excludes a list of predetermined terms has no chance of working properly in the long run. Moreover, these systems originate first for the English language: if they are to be used for a different language, the list of words must be translated, with additional human intervention, since attempts made with automatic translators have not proved up to the task."
Together with two colleagues from the Department of Computing Sciences (Dirk Hovy and Giuseppe Attanasio) and a Professor from the Polytechnic University of Turin (Elena Baralis), Dr. Nozza developed Entropy-based Attention Regularization (EAR), a tool capable of mitigating this kind of bias without the use of a list of word. It also works in any language, provided, of course, that the training phase of the hate speech detection system was carried out in that language.
The key is the request, made to the system, to pay less attention to individual terms (all individual terms, not just those included in any list) and more attention to context. "Our system manages to reduce the bias significantly, with a performance comparable to other techniques in terms of hate speech detection," Nozza concludes.