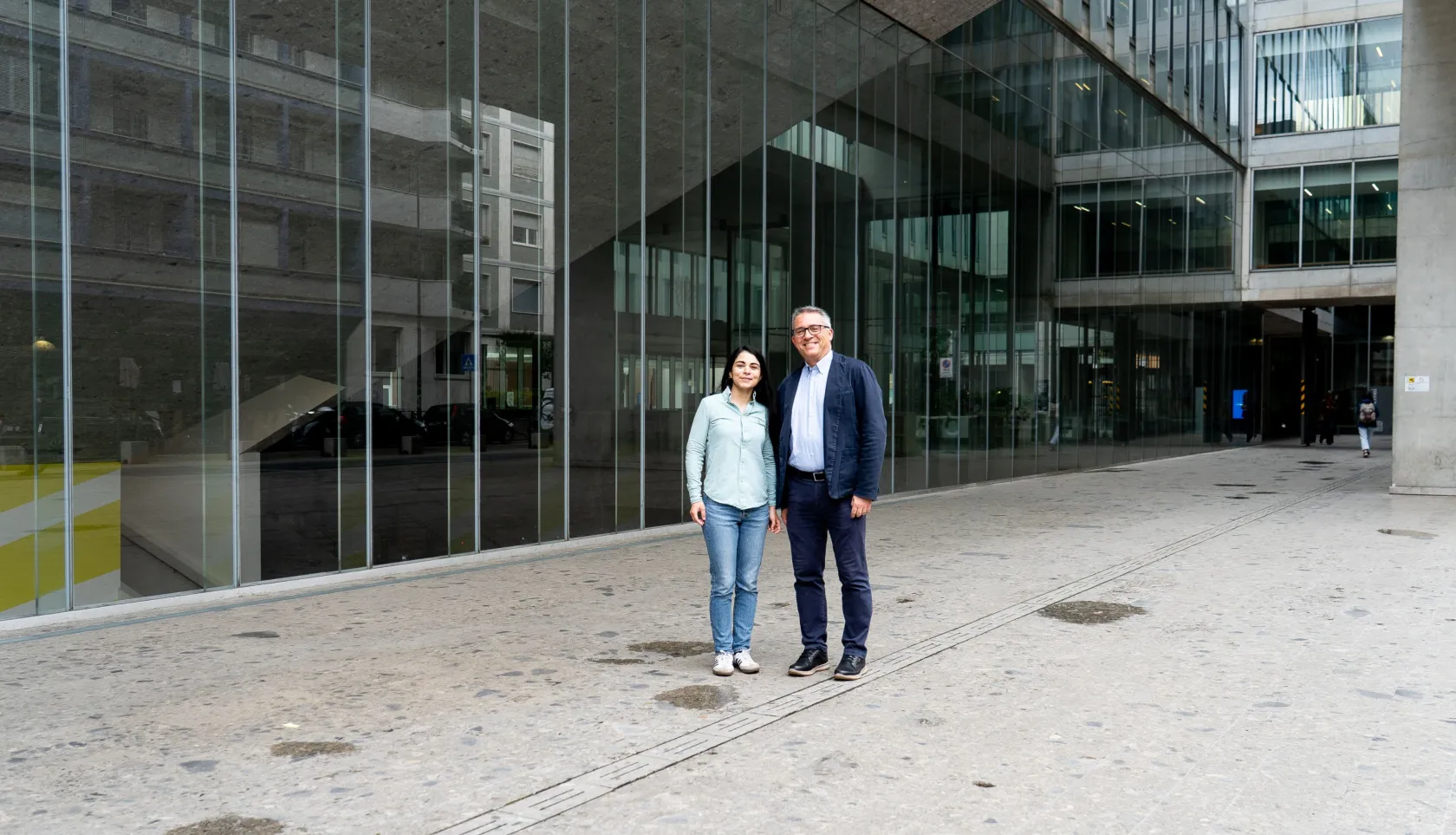
Cryptocurrencies, Between Apparent Chaos and Predictable Signals
Cryptocurrencies are often considered highly speculative assets, with erratic courses hard to connect to observable economic fundamentals. However, recent work by Massimo Guidolin and Serena Ionta of Bocconi University challenges this perception, showing that certain blockchain characteristics and sentiment measures can predict, with some reliability, the future returns of a large set of cryptocurrencies.
It is precisely this hypothesis that the new working paper by Massimo Guidolin and Serena Ionta, Predictive Sorting of Cryptocurrencies Based on Fundamentals and Sentiment, studies. The authors develop a quantitative approach that uses the factor-mimicking portfolios (FMP) technique to transform non-tradable variables such as hashrate, number of active users and Google searches, into empirically testable factors. This allows them to assess the relationship between technical fundamentals, behavioral dynamics, and cryptocurrency performance in an asset pricing framework.
The context: volatility and uncertainty
After years of expansion and as many episodes of instability, the cryptocurrency market is still a maturing financial environment marked by high volatility and a strong speculative component. Despite the now systemic size of the industry, price predictability remains an open question for scholars and investors.
Guidolin and Ionta's study fits into this debate, aiming to test whether underlying dynamics of blockchain technology, particularly network growth and computational intensity, can be predictive determinants of returns. The sentiment component, which is measured in the study using Google Trends-based indicators, serves as a proxy for aggregate market expectations, which are hard to observe directly, and is included in the framework of explanatory factors for cryptocurrency returns.
The method: between numbers and perceptions
The analysis is based on a weekly dataset covering 41 cryptocurrencies over the period 2015-2022. Three main indicators are set up: one related to the computational power of the network, one to the number of active users, and one to investor sentiment. The central methodological contribution is the use of FMPs, synthetic portfolios that make it possible to transform variables that are not directly observable in the market into empirically testable factors in the asset pricing framework. An additional innovative element is the systematic adoption of out-of-sample (OOS) analysis, which allows the predictive effectiveness of models to be assessed outside the sample used for estimation. This perspective, the authors point out, is crucial for distinguishing purely explanatory models from those that are potentially useful in real-world forecasting applications.
Impacts: insight turned into strategy
The next step is to translate this predictability into investment strategy. This is where portfolio sorting comes in: each week, cryptocurrencies are ranked according to their predictability (as measured by a measure of model fit to the data, the OOS R-squared or, alternatively, through the Certainty Equivalent Return - CER). The most predictable are bought, the least predictable shorted. The result? Significant and consistent extra-returns, with performance exceeding even that of portfolios constructed on a purely statistical basis. The CER, in particular, is a metric that accounts for risk aversion: it quantifies how much an investor would be willing to pay to access a certain predictive model. In other words, it measures not only the effectiveness, but also the economic utility of predictions. “It's an approach that allows us to move from pure academic exercise to concrete implications for active portfolio management,” Guidolin notes.
Toward a more rational crypto finance?
The study contributes to the debate on the predictability of returns in the cryptocurrency market by showing that factors attributable to technology fundamentals and sentiment indicators can be systematically associated with price dynamics. Empirical evidence suggests that such signals, appropriately modeled through mimetic portfolios, can be integrated into active forecasting and allocation strategies, improving information efficiency within portfolio management.
In this context, the proposed approach makes it possible to reduce the apparent randomness of crypto markets by providing quantitative tools for a more structured reading of their evolution. As Guidolin points out, the goal is not to eliminate uncertainty, but to learn how to manage it through more robust models.