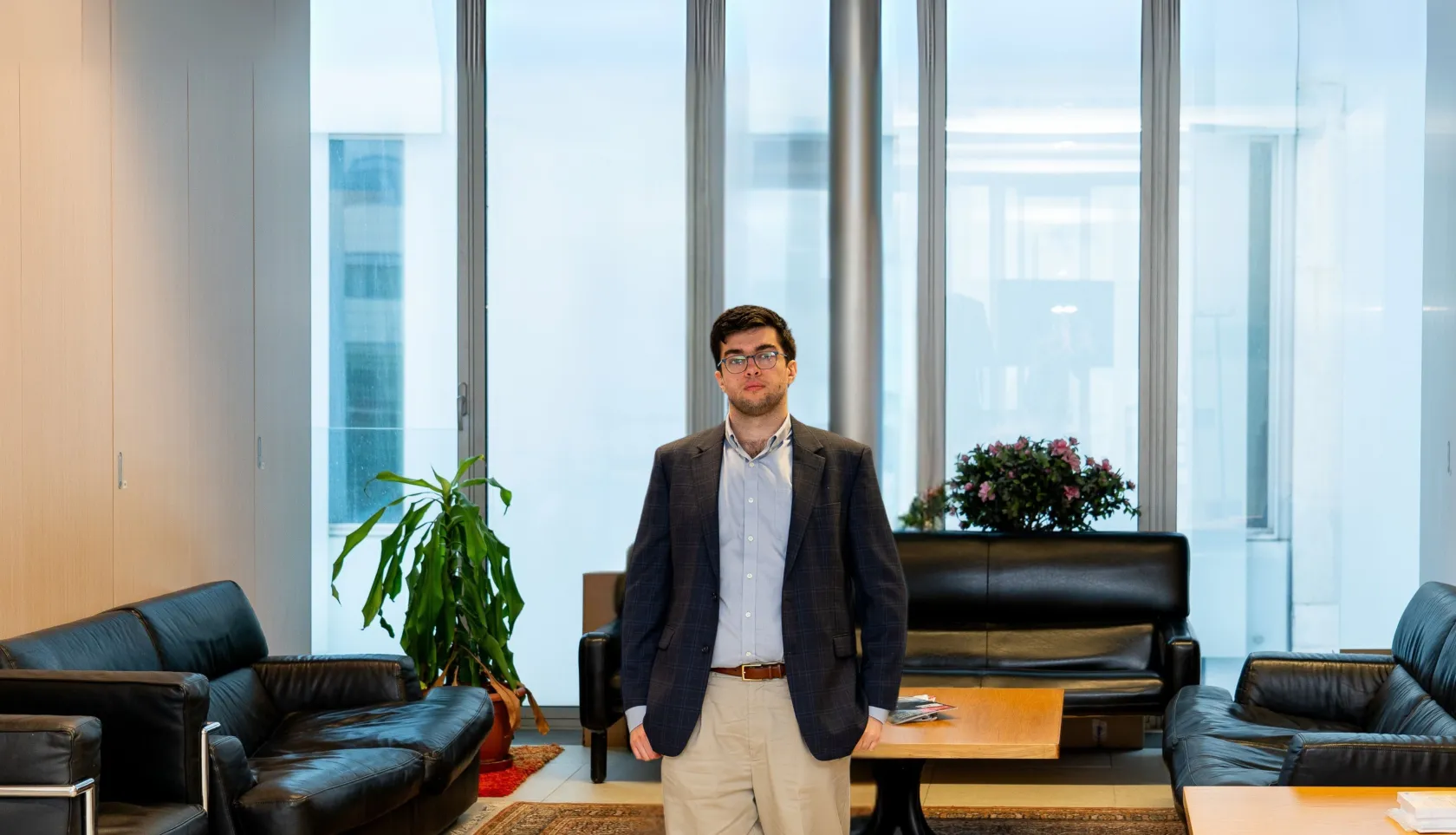
Cracking the Code of Human Choice
When economists try to understand human behavior—whether it’s buying groceries, choosing a movie, or casting a vote—they often rely on something called the random utility model. The idea is elegant: people act to maximize their satisfaction, but their preferences can vary randomly from moment to moment.
Turning this appealing theory into something you can actually test with real-world data, however, has proven to be extremely difficult. The most influential method, developed by Kitamura and Stoye in 2018, made a major leap forward. It allowed economists to test random utility models without assuming anything about the population’s tastes. But as powerful as it was, their method had a fatal flaw. As Christopher Turansick (Bocconi Department of Decision Sciences and IGIER research center) notes in his new study, “the test is subject to computational constraints which leaves execution… infeasible in many applications.” When the number of choices gets large—a common situation in real life—the calculations required balloon to the point of being practically impossible.
A fresh perspective: outlines, not details
In his 2025 paper, An alternative approach for nonparametric analysis of random utility models, published in the Journal of Economic Theory, Turansick offers a fresh and remarkably simple solution to the problem. Instead of describing choice behavior by listing every possible way a person could decide (what the old method did), he suggests focusing on the boundaries of rational behavior.
It’s a bit like trying to map a mountain range. You could laboriously chart every rock, tree, and slope—or you could sketch the silhouette and still capture the essence. Turansick’s insight is that by working with the boundaries (technically, the “faces” of a geometric object called a polyhedral cone) rather than all the intricate possibilities (“vertices”), one can create a much leaner, faster test of the random utility hypothesis.
This change isn't just cosmetic. It dramatically reduces the computational burden, making the test feasible even when there are many choices. As Turansick puts it, “our methodology is not only theoretically implementable, but also implementable from an econometric perspective.” In other words, it doesn't just sound good—it actually works in practice.
Redefining rationality
Turansick’s approach also brings a deeper conceptual shift. Traditional methods needed to peek inside the black box of human preferences, reconstructing every possible order of choices to test rationality. His method turns that around. Instead of trying to describe every internal preference, it checks whether the observed choices could be extended logically without running into contradictions.
This leads to a new way of defining rational behavior—one that doesn't depend on mapping out every hidden preference. “Our new axiom can be stated without reference to the model,” Turansick explains. Rationality, in his framework, becomes a question of feasibility rather than introspection: could the observed behavior exist within a system that respects certain logical boundaries?
Why this matters
Making the random utility model faster and more practical to test might sound like a technical tweak. In reality, it could transform how economists—and other social scientists—study behavior. Until now, even modestly complicated datasets often made proper testing impossible. Turansick’s approach, on the other hand, might help researchers apply rigorous, data-driven analysis to complex, real-world choices: online shopping habits, political voting patterns, healthcare decisions, and much more.